
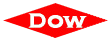




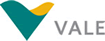

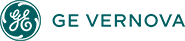




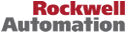

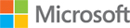
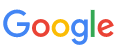

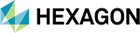


Keywords: Industrial Data Platform, Business Intelligence, Statistical Process Control, Overall Equipment Effectiveness, No Code, Low Code, Advanced Analytics, Enterprise Manufacturing Intelligence, ARC Advisory Group.
What is the best way to compare industrial analytic software solutions? Several compelling solutions are available on the market, so a good way to compare them is by their functionality. These analytic platforms, sometimes known as industrial data platforms, provide a tool set for businesses to absorb, organize, discover, and analyze data to reveal actionable insights that can help improve decision-making and inform business strategy.
Many different industrial data platforms are available, each with its own unique set of features and capabilities. Some of the most important modules that you might find in industrial analytics software include:
Predictive Maintenance: Use machine learning algorithms to predict when equipment is likely to fail, allowing maintenance teams to take proactive measures to prevent downtime and reduce costs.
Quality Control: Ensure products meet quality standards by analyzing data from sensors and other sources to identify defects and other issues.
Supply Chain Optimization: Optimize supply chains by analyzing data from suppliers, logistics providers, and other sources to identify inefficiencies and opportunities for improvement.
Energy Management: Reduce energy consumption and costs by analyzing data from sensors and other sources to identify areas where energy usage can be optimized.
Process Optimization: Optimize production processes by analyzing data from sensors and other sources to identify bottlenecks, inefficiencies, and other areas for improvement.
These are just a few examples of the many different modules that might be included in industrial analytics software. The specific modules that are most important for a particular organization will depend on the unique needs and goals.
Industrial managers today are under pressure to optimize production processes and make them more efficient. This is the starting point for thinking about the use of industrial data to increase efficiency. Yet, when this idea is further elaborated, one quickly comes to the realization that there may not be enough data available to achieve this goal.
Many managers realize that, in practice, it is not easy to access important data. While it may be easy to access data in automation systems, access to laboratory data, alarm data, shift logbook data or IoT sensor data can be arbitrarily complex and error prone.
Assuming that access to relevant company data is available, the next step managers face is the challenge of contextualizing existing data. This means that timestamps and information on the origin of the data must be linked to the process data - the only way to turn raw data into valuable process data. This contextualized data then forms the basis for data analysis, which can help increase the efficiency of company processes.
Once the type of raw data and the required contextualization have been clarified, the implementation of the "efficiency improvement" project begins. It is often assumed that the company's own IT department or an external service provider will provide access to the raw data. Inhouse software should contextualize raw data and make it available as process data on a data platform. This approach, the cooperation of the company's internal IT with an external service partner, appears to be suitable because the required investment is low. However, this may be a mistake, as this type of project often takes too long, and it may be difficult to keep the resulting data platform up to date over its lifecycle. The result is that a low-cost project turns into an expensive and error-prone solution when viewed over the lifecycle of an industrial plant.
It is therefore recommended to use solutions available on the market for industrial data platforms. The advantages of using this solution are easy to summarize:
Providers offer training and support for their solutions
Standardized documentation is available for the entire platform
System integration is realized globally by service partners
Hardware and software are constantly being further developed
Solutions have already proven their efficiency in many industries
Data exchange with industrial communication protocols is proven in operation
Project implementation can be done with a scheduled approach
The user can choose between different payment models
Perhaps the most important advantage, however, is that the individual project participants remain focused on their core competencies. The IT department is responsible for the availability of company data, while the OT department focuses on production processes and uses up-to-date data analysis for process optimization. A system partner integrates the data platform with the necessary interfaces in the OT area and trains the system users.
To implement industrial data analytics to support sustainability, it is recommended to follow these steps:
Identify the sustainability goals in the organization and the areas where data analytics can help achieve them. This could include reducing energy consumption, minimizing waste, or improving supply chain efficiency.
Collect relevant data from various sources such as sensors, machines, and other systems. This data could include energy consumption, water usage, carbon emissions, and other environmental metrics.
Clean and preprocess the data to ensure accuracy and consistency. This could involve removing outliers, filling in missing values, and normalizing the data.
Analyze the data using various techniques such as statistical analysis, machine learning, and predictive modeling. This will help you identify patterns and trends in the data that can be used to optimize operations and reduce environmental impact.
Visualize the result of your analysis using charts, graphs, and other visualizations. This will help you communicate your findings to stakeholders and identify areas for improvement.
Implement changes based on your analysis to improve sustainability performance. This could include optimizing processes, reducing waste, or investing in renewable energy sources.
Monitor and evaluate the impact of your sustainability initiatives over time. This will help you identify areas for further improvement and ensure that you are meeting your sustainability goals.
By following these steps, you can leverage industrial data analytics to support sustainability and drive positive environmental impact.
As mentioned, it is not easy to generate valuable data from raw company data to increase efficiency. This is where experts from within the company should work closely with external system partners for data platforms. The first step is to define which raw data is to be used. The usual sources of raw data are:
Data from the automation systems (OT)
Data from company IT (IT)
Data from additional IoT sensors (IOT)
Data from cloud solutions (cloud)
These data are often compiled using manual entries. This is necessary if the automatic data transfer does not work. Typical examples are data from the "shift book" or data generated during maintenance. Additional data is often used to contextualize and supplement the raw data. Another important aspect is the analysis of error root causes. Here, manually entered data can be used for an anomaly analysis of the process flows.
To supply the industrial data platform with raw data, access to the various data sources is necessary. If we now analyze the various systems in the company, we can extract data from systems and devices such as PLC, DCS, field devices and IoT sensors, and software such as ERP, MES, LIMS and CMMS.
To better understand the comparability and the cause-and-effect principle of the data, the clear contextualization of these different raw data is essential. In the end, fact-based decisions should be made in relation to the production processes. To do this, the raw data must be prepared and processed accordingly. At this point, it also becomes clear that this data preparation must be carried out by service providers with the appropriate expertise.
While industrial data platforms software offers numerous benefits, there are several reasons why some businesses might not implement or use it:
Cost: Data platforms can be expensive. The costs include not only the price of the software itself, but also the infrastructure needed to support it, the cost of training employees to use it, and ongoing maintenance and support costs.
Complexity: Data platform tools can be complex and require a certain level of expertise to use effectively. This can be a barrier for smaller businesses or those without a dedicated IT team.
Data privacy concerns: Businesses dealing with sensitive information might be hesitant to use data platforms due to concerns about data privacy and security.
Resistance to change: Like any new technology, there can be resistance to implementing data platforms. This can come from employees who are comfortable with current processes and wary of change.
Lack of awareness: Some businesses might not be aware of the benefits of data platforms, or they might not understand how it applies to their operations.
Time-consuming implementation: Implementing a data platform can be a lengthy process, requiring a significant time investment.
It's important to note that while these challenges exist, they can often be overcome with careful planning, adequate training, and the selection of the right data platform tool that fits the business's needs and capabilities.
Some data platforms require IT implementation to build the analytical environment, connect necessary data sources, and help prepare data for usage; others are designed to be primarily configured and used by non-expert users, without the help of IT for deployment (known as self-service). Business and data analysts, data scientists, or other business stakeholders can utilize this software to prepare, model, and transform data to better understand the day-to-day performance of the company and make informed decisions.
Fundamentally, for a product to be categorized as an analytics platform it must be an end-to-end analytics solution, which incorporates five elements: data collection, data storage, data structuring/processing, data visualization, and data analytics. Although standalone data preparation tools exist that assist in the process of discovering, blending, combining, cleaning, and enriching data—so large datasets can be easily integrated, consumed, and analyzed—analytics platforms must incorporate these functionalities into their core offering. Analytics platforms must support data blending and data modeling, giving the end user the ability to combine data across different databases and other data sources and allowing the end user to develop robust data models of this data.
The reports, dashboards, and visualizations created using analytics platforms can break down data to a granular level, depict connections and trends between multiple datasets, and create data visualizations that make the data easier to understand for non-expert stakeholders. Products that only provide the visualization component are categorized as data visualization software, which includes products primarily designed to create charts, graphs, and benchmark visualizations. Some analytics platforms offer embedding functionality to place dashboards or other analytics capabilities inside applications; these products are considered embedded analytics software.
Products specifically designed for ingesting and integrating big data clusters are categorized as big data analytics software. Other features of analytics platforms can include natural language search functionality and augmented analytics. Natural language search refers to the ability to query data using intuitive language, frequently in the form of a question. Augmented analytics refers to the process of using machine learning for deriving insights from the data and supporting non-expert users in working with and visualizing data, such as automated data preparation and discovering hidden patterns in the data.
There are many factors to consider when choosing a data platform for your organization. Some common selection criteria are:
Fit with business process type: The data platform should match the type and complexity of the business processes that need to be analyzed and improved. For example, if the organization needs to monitor and optimize workflows, you might look for software that supports business process management (BPM) features.
Process design and deployment: The data platform should allow to design and deploy custom data models, reports, dashboards, and analyses, or provide readymade templates and solutions. Consider who will be responsible for developing and maintaining the software, and how easy it is to use and learn.
Integration: The data platform should be able to access and integrate data from various sources, such as databases, data warehouses, cloud services, or web pages. Check how the software handles data quality, security, and governance issues.
Performance management: The data platform should help to measure and improve the performance of business processes, using techniques such as key performance indicators (KPI), balanced scorecards, benchmarks, and alerts. You should also look for software that supports predictive and prescriptive analytics, which can help you forecast and optimize future outcomes.
Out-of-the-box value: The data platform should offer a good return on investment (ROI) and a low total cost of ownership (TCO). Compare the features and capabilities of different software products, as well as their prices, licensing models, and support services.
These are some of the main selection criteria for data platforms, but there are many more that you can use depending on specific needs and preferences of the organization.
Industrial data platforms are software solutions that help industries collect, process, analyze and visualize data from various sources to optimize their operations, performance, and profitability.
Industrial data platforms optimize among other things the following industrial processes: predictive maintenance, quality control, supply chain optimization, energy management and process optimization. However, they also face challenges, such as cost, complexity, privacy concerns, resistance to change and time-consuming implementation.
It is important to choose an industrial data platform that suits a company’s needs. This statement can easily be misunderstood, specifically the fit of the industrial data platform with the type of business processes, process design and implementation, integration, performance management and user acceptance of the solution. Another important criterion is the implementation time in the organization. This includes integration into the existing OT and IT environment, as well as time required for user training.
The following table illustrates the evolution of various software concepts. This is because the collection and processing of industrial data has evolved since 1990. The table is a simplified overview from “historian software” to the modern “data platform.” Functionalities and possibilities of data evaluation are presented here in tabular form.
If a business is looking for a solution for process optimization, then the following key points may be significant for the decision-making process:
1) The software provider should have references in the industry. It is important that the provider and the user have the same understanding of the optimization applications.
2) Operator acceptance of the software solution is critical. The use of an industrial data platform is a transformation project in the organization. Operators reject software solutions that they cannot manage themselves. If an expert must be consulted first for every new graphic or dashboard, acceptance will be low. This is also a cost factor, as the time for an expert costs money. It is therefore important to ensure that users can operate the software themselves and adapt it to the company's processes after a brief introduction. Apps in the world of mobile phones are a good model for this.
ARC Advisory Group clients can view the complete report at the ARC Client Portal.
Please Contact Us if you would like to speak with the author.
Obtain more ARC In-depth Research at Market Analysis